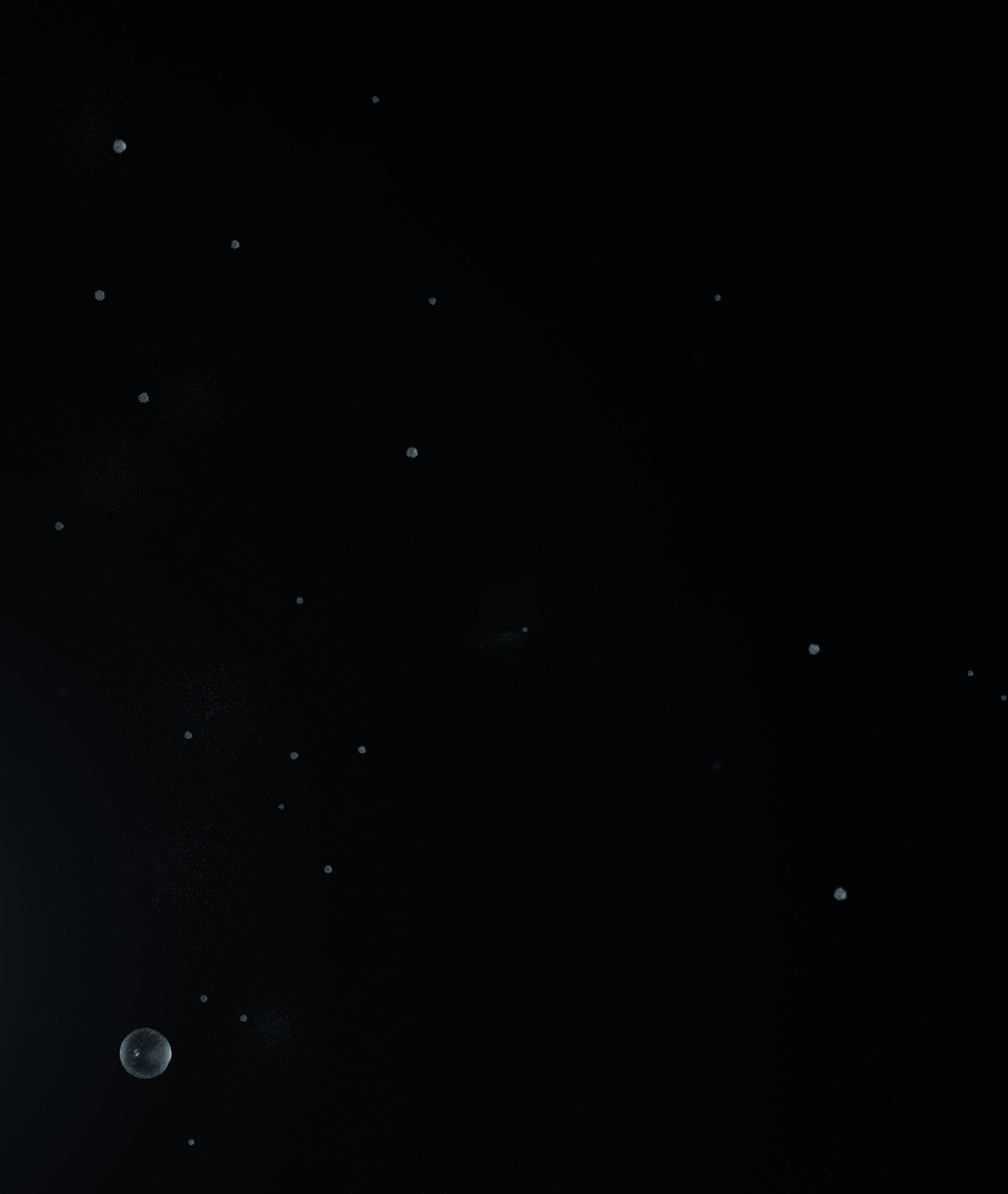
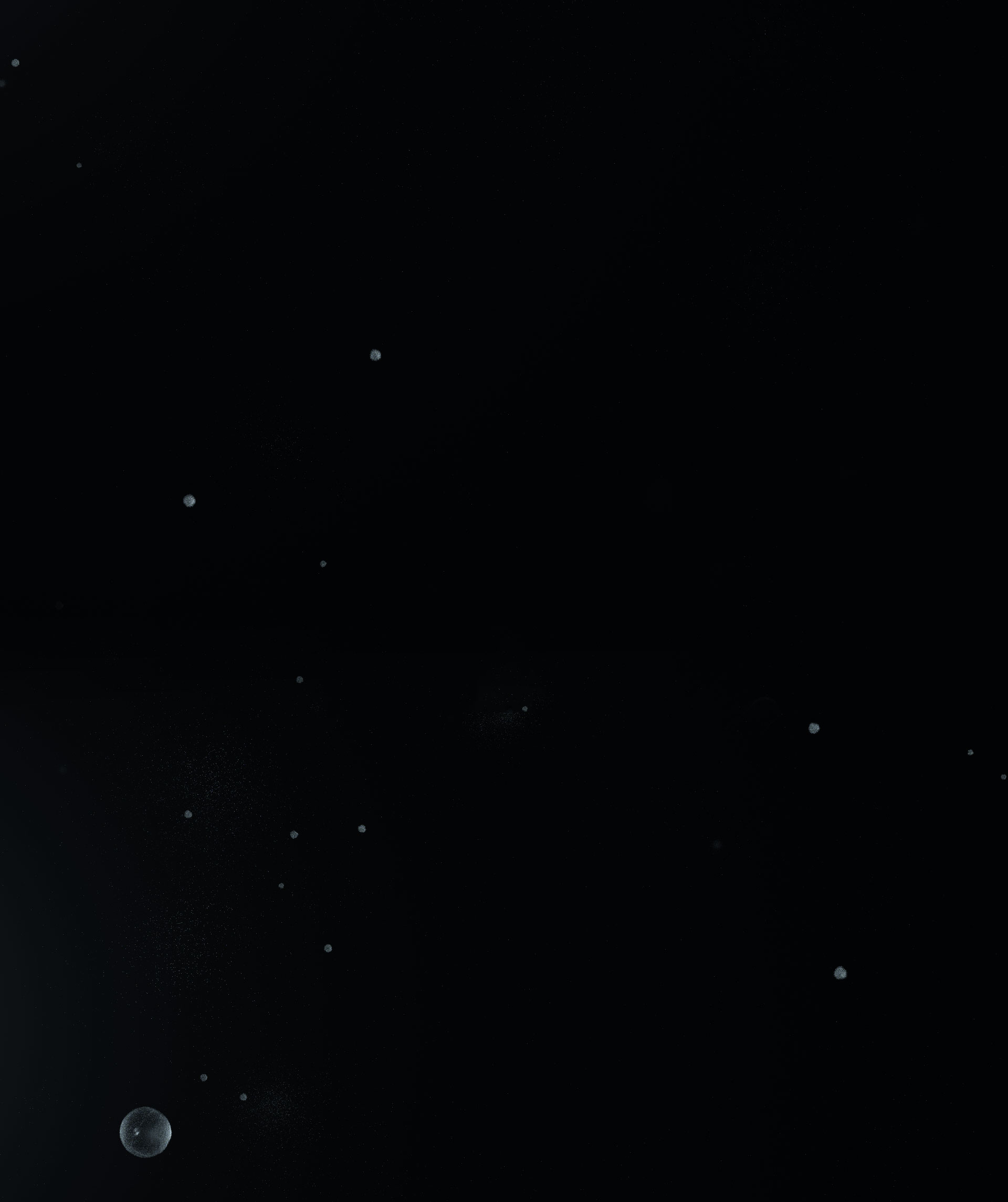
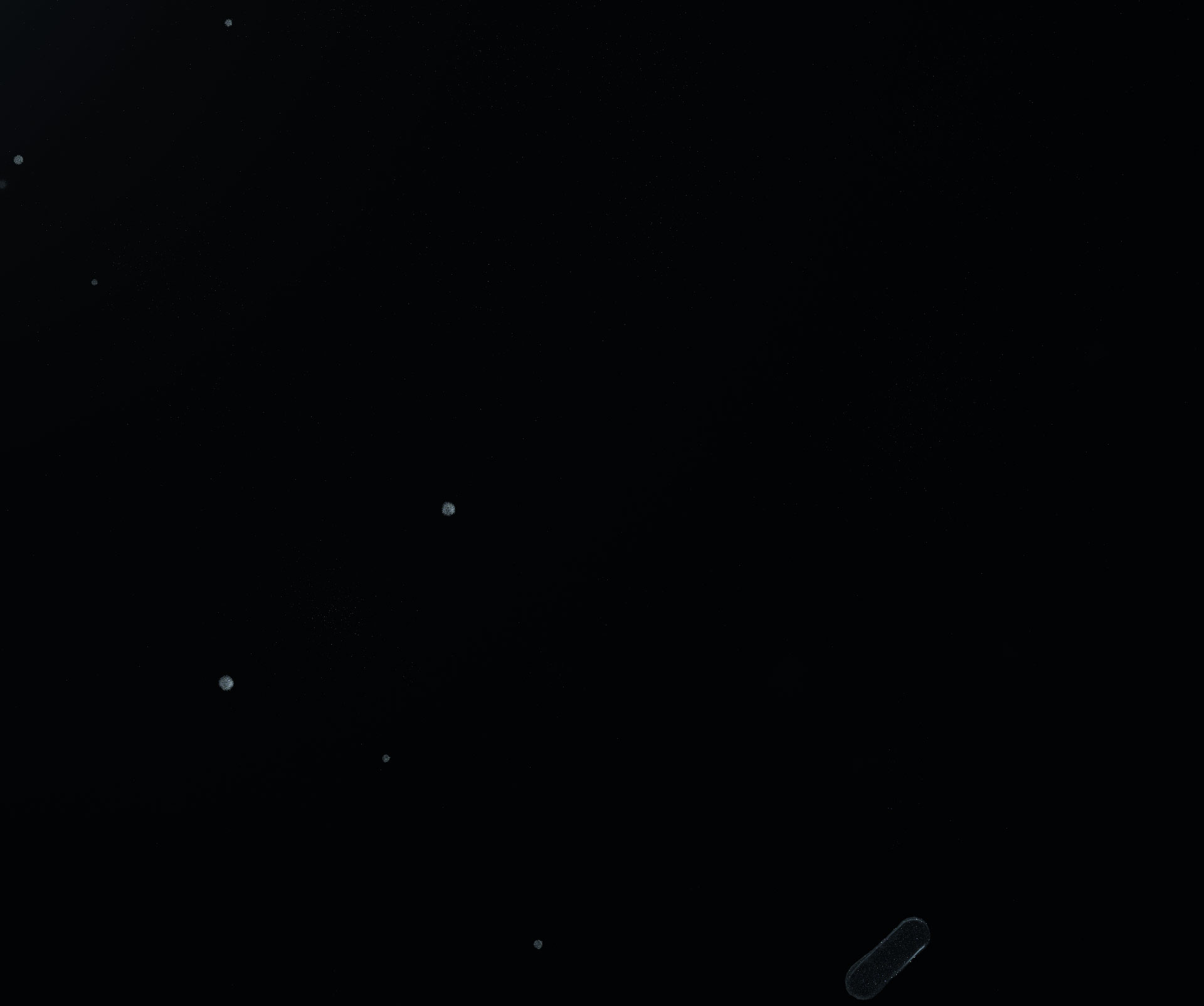
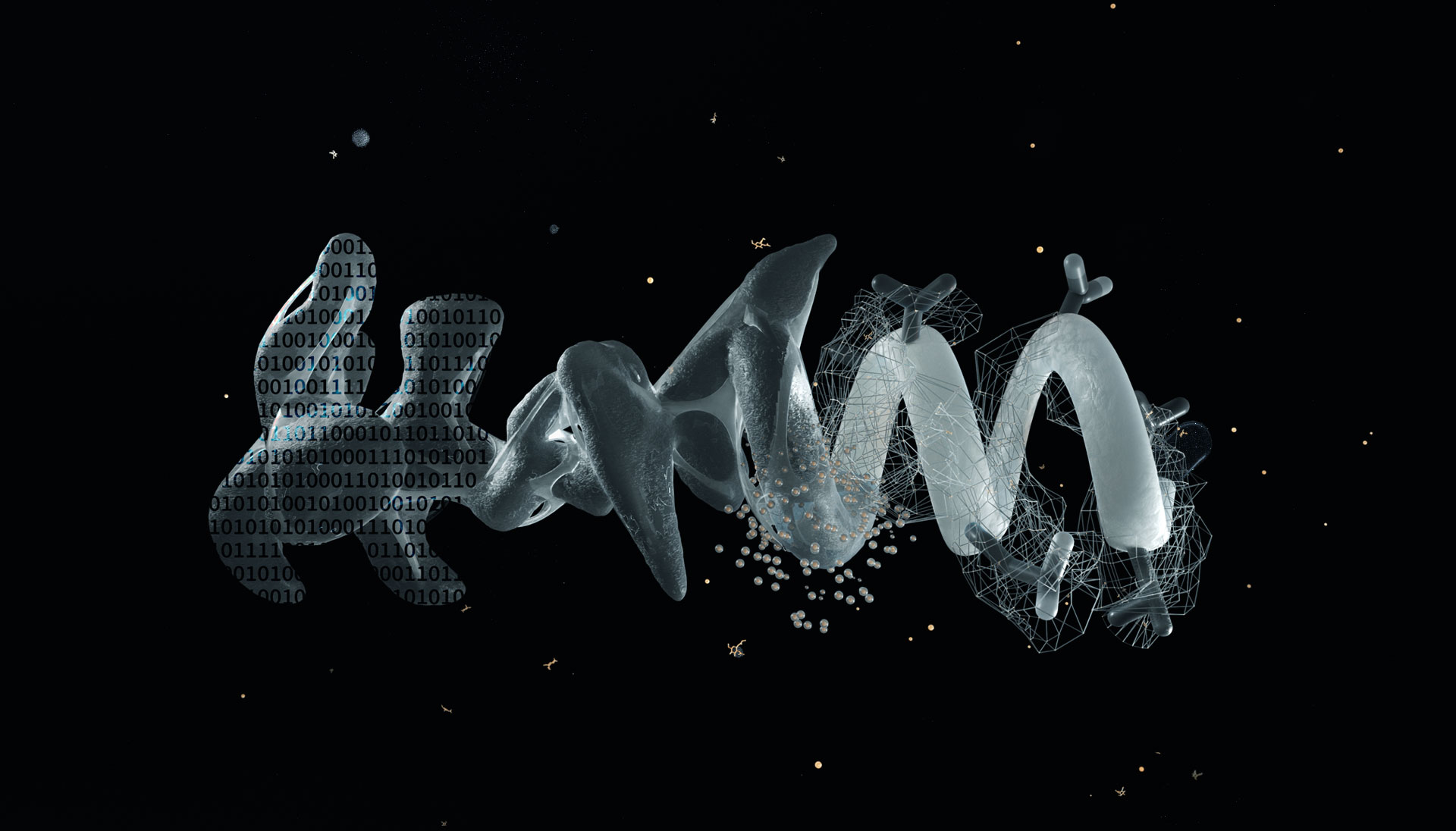
TECHNOLOGY - CryoNet
B A C KCryo-EM Modeling
with Deep Neural Network
We are developing a new differentiable neural network based method (CryoNet) to directly identify the 3D atomic model from cryo-EM density map in a few hours without any human interference.
Model building remains a bottleneck as the last step in structure determination, although the progress is becoming more and more automated.
Manual operation is time-consuming and inevitably error-prone. Significant incorporation of automation has resulted in the ability to obtain near-atomic resolution reconstructions within hours to days. However, molecular model building is difficult to automate since it relies substantially on human expertise, which limits scalability for use by wider community. A model that represents the atomic coordinates of each amino acid is constructed and fitted into the 3D electron density volume as the final step. Specific amino acids to a density volume are manually assigned by structural biology experts with the help of 3D visualization tools. Attempts to automate this process are far from satisfactory given to the accuracy, coverage, time-consuming and requirement of human intervention.
AI-driven: faster and more accurate than existing automated solutions without any human intervention.
As part of the push to improve efficacy and performance of model building, We proposed a learning-based method from a totally novel perspective. The power of deep neural network is leveraged to develop a novel framework for end-to-end atomic model building from Cryo-EM density map.
These endeavor for better model building is expected to fuel the rapid growth of cryo-EM atomistic structures. We hope our exploration will facilitate the integration of machine learning and more collaboration with experts from fields outside of traditional structural biology.
● This is the first attempt to formulate molecular structure determination from Cryo-EM density volumes with a deep learning approach.
● We adapt a novel 3D network architecture for atomic model building in density volumes, and proposed a Cryoformer block for better performance.
● We carefully designed a way to bridge Cryoformer and AlphaFold to achieve Cryo-EM density-based protein complex structure prediction.